Introduction
In the pharmaceutical industry, dissolution testing is critical for drug development, quality control, and regulatory submission. It measures the rate and extent of drug release from a dosage form, which directly impacts the drug’s bioavailability and therapeutic efficacy. Currently, dissolution testing is routinely performed using standard dissolution vessels under controlled conditions. However, this approach is time-consuming, labor-intensive, and destructive. Therefore, as the pharmaceutical industry transitions toward continuous manufacturing (CM), it has become increasingly relevant to implement real-time release testing (RTRt) for dissolution.
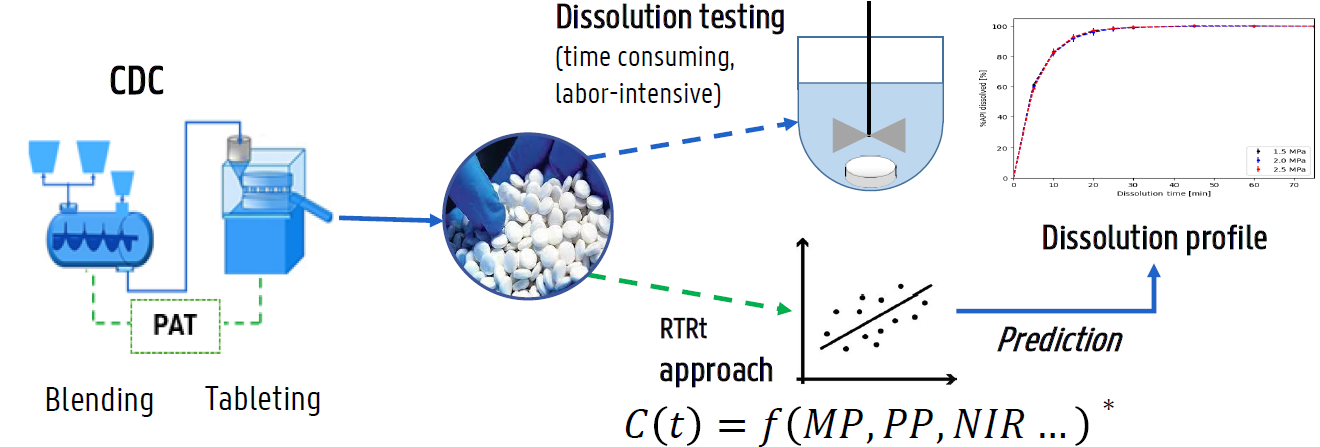
Real-time release testing (RTRt) is a system that enables real-time assurance of product quality based on process data. Compared to conventional testing, RTRt is nondestructive, offering a more efficient and sustainable approach to accelerating drug development and reducing product lead time.
Advances in Process Analytical Technology (PAT) tools, such as Near-Infrared (NIR) spectroscopy and chemometrics, have been recognized as key enablers of RTRt implementation. For solid dosage forms, NIR combined with Partial Least Squares (PLS) regression has been widely evaluated by various researchers for the development of predictive dissolution modeling (PDM) to ultimately enable RTRt in CM. However, the approach to developing PDM varies between studies, and no single, universal strategy currently exists. As the field matures, establishing a comprehensive framework for PDM development may be essential to support broader adoption of RTRt within the pharmaceutical industry.
Study design/Methodology
This study presents a comprehensive predictive dissolution modeling (PDM) framework applicable to real-time release testing (RTRt) of immediate-release tablets manufactured via continuous direct compression (CDC). The formulation was composed of a poorly soluble API combined with three filler combinations (MCC/DP, Mannitol/MCC, and Lactose/MCC) and varying levels of croscarmellose. These filler combinations, previously reported to exhibit different disintegration mechanisms, were selected to ensure that the PDM captures a range of disintegration behaviors.
Formulations were processed using a Consigma® CDC-50 (GEA) system, followed by tableting on a MODUL S (GEA) tablet press. Additionally, in-line NIR spectra were collected in the feed frame of the tablet press using a SentroPAT FO system equipped with a SentroProbe DR LS.
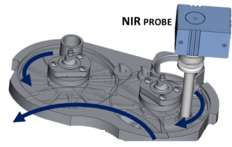
Development of the predictive dissolution framework:
The PDM was developed using the Weibull model and three PLS regression models. First, the Weibull model was used to fit the dissolution profiles, from which the parameters a (scale) and b (shape) were determined. PLS models were built to separately predict the a and b parameters using material properties (12 predictors) and compression force as inputs. A third PLS model was developed to predict the potency factor (C₀) using in-line NIR spectra collected from the tablet press feed frame. The outputs of the three PLS models were combined to predict the complete dissolution profiles of the tablets. The performance of the combined model was evaluated using the coefficient of determination (R²), and all three models were validated using 7-fold cross-validation
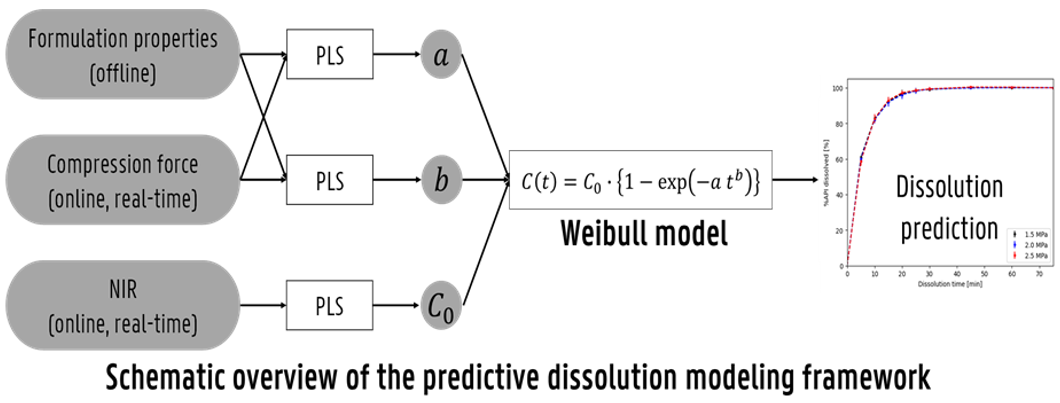
Key Findings:
MCC/DCP-based formulations were less influenced by disintegrant level and compression force compared to Mannitol/MCC- and Lac/MCC-based formulations. Blend properties such as high vapor sorption and low solubility were associated with a faster initial dissolution rate. Dissolution profiles were successfully predicted with reasonable accuracy, achieving an average R² of 0.94 for MCC/DCP and Mannitol/MCC – based formulations, and 0.89 for Lac/MCC – based formulations.
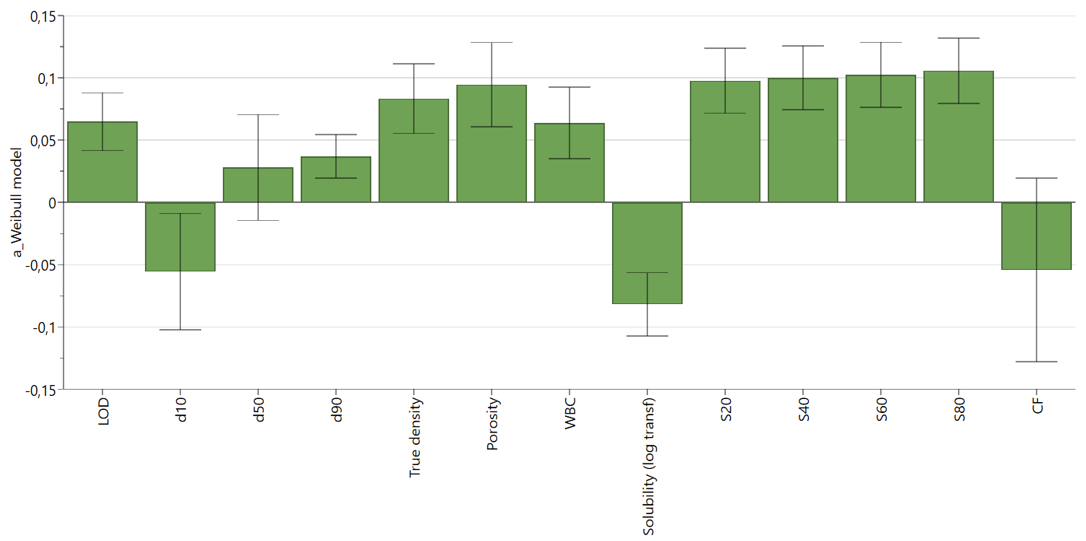
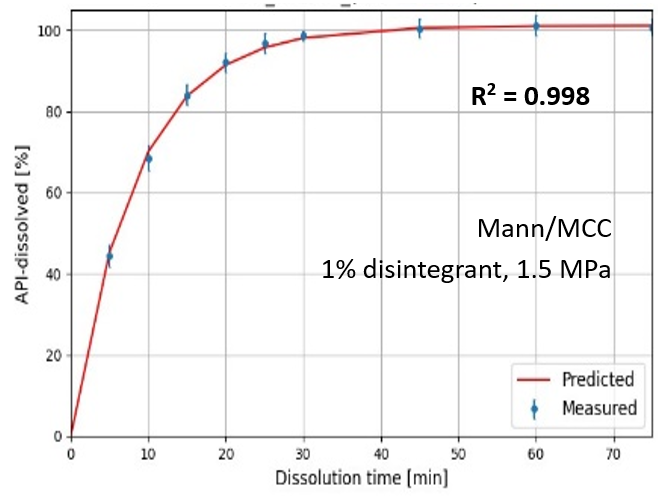
Overall, this study serves as an initial step toward developing a comprehensive RTRt platform for dissolution prediction, ultimately aiming to replace time-consuming dissolution testing. The findings suggest that the proposed PDM framework could be applicable to a wide range of formulations, operational settings, and dissolution conditions.
Feel free to contact the author or explore additional information in a poster presented at international conferences.